Mercilina Norman
The healthcare industry is undergoing a profound transformation driven by technological advancements that promise to enhance patient care, streamline operations, and improve outcomes. In 2024, several groundbreaking innovations are making headlines and reshaping the future of healthcare. This article delves into the latest trends in healthcare technology, exploring their implications, challenges, and potential to revolutionize the industry.
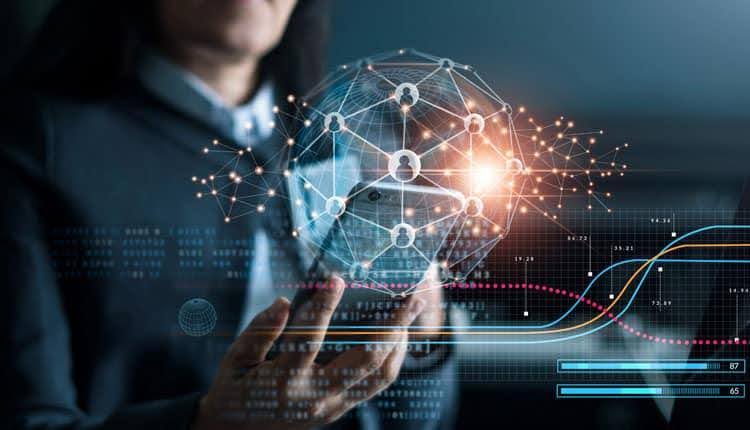
Generative AI in Drug Discovery
Understanding Generative AI
Generative AI models are revolutionizing drug discovery by creating novel compounds and predicting their interactions with biological targets. Traditional drug discovery processes are notoriously time-consuming and expensive, often taking over a decade and billions of dollars to bring a new drug to market. Generative AI offers a promising solution by significantly accelerating this process.
Generative AI involves algorithms that can generate new data samples that resemble the training data. In drug discovery, these models can create new molecular structures with desired properties. Techniques like Generative Adversarial Networks (GANs) and Variational Autoencoders (VAEs) are commonly used in this context.
Impact on Drug Development
By simulating countless molecular interactions in silico, generative AI can identify promising drug candidates much faster than traditional methods. This accelerates the initial phase of drug discovery, where potential compounds are identified and synthesized. Companies like Insilico Medicine and Atomwise are at the forefront of using generative AI to discover new drugs for conditions such as cancer and neurodegenerative diseases.
Challenges and Considerations
Despite its promise, generative AI in drug discovery faces several challenges. One major issue is the quality and representativeness of the training data. Poor data quality can lead to inaccurate models and ineffective drug candidates. Additionally, the integration of AI-generated compounds into existing drug development pipelines requires extensive validation and regulatory approval, which can be complex and time-consuming.
Future Prospects
The future of generative AI in drug discovery looks promising, with ongoing advancements in AI algorithms and computational power. Collaboration between AI companies, pharmaceutical firms, and regulatory bodies will be crucial in overcoming current challenges and fully realizing the potential of this technology.
AI-Driven Predictive Analytics in Pandemic Response
AI in Pandemic Prediction
The COVID-19 pandemic underscored the need for robust predictive analytics in managing health crises. AI-driven models are now being developed to predict and manage future pandemics, offering a proactive approach to public health. Predictive analytics involves using historical data to make informed predictions about future events. In the context of pandemics, AI models analyze vast amounts of data, including epidemiological, demographic, and social media data, to identify patterns and predict disease outbreaks.
Applications and Success Stories
During the COVID-19 pandemic, AI models developed by companies like BlueDot and HealthMap successfully predicted the initial outbreak in Wuhan, China, days before official reports. These early warnings allowed for more timely public health interventions. Moving forward, AI-driven predictive analytics can play a crucial role in monitoring emerging infectious diseases and mitigating their impact.
Challenges and Ethical Considerations
The use of AI in pandemic response raises several challenges and ethical considerations. Data privacy is a significant concern, as predictive models often require access to sensitive personal information. Ensuring data security and protecting individuals’ privacy are paramount. Additionally, the accuracy of AI predictions depends on the quality and completeness of the data, which can vary across regions and populations.
The Road Ahead
The future of AI-driven predictive analytics in pandemic response is bright, with ongoing advancements in data collection and analysis techniques. Collaboration between governments, healthcare organizations, and technology companies will be essential in creating robust and ethical predictive models. These models have the potential to transform global health security by enabling proactive and data-driven responses to emerging health threats.
Digital Twins in Healthcare
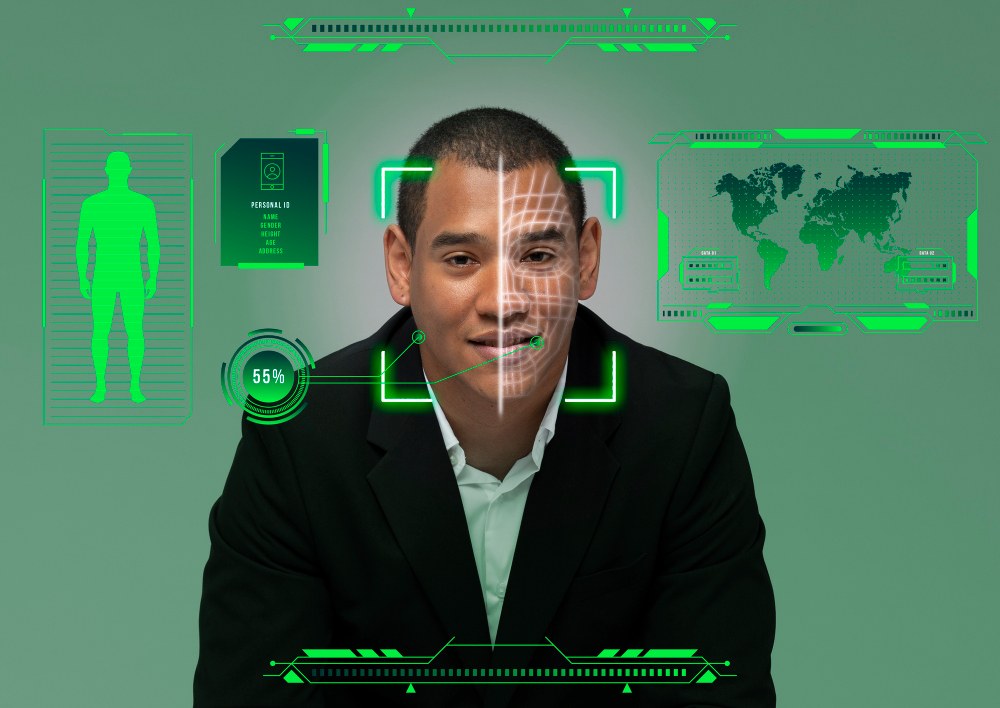
What Are Digital Twins?
Digital twin technology, which creates virtual replicas of physical entities, is making its way into healthcare. By simulating patient-specific models, digital twins allow for personalized treatment planning and real-time monitoring. This approach enhances precision medicine, enabling healthcare providers to tailor treatments based on individual patient data.
A digital twin is a virtual representation of a physical object or system, continuously updated with real-time data. In healthcare, digital twins can be created for patients, medical devices, or entire hospital systems. These digital replicas simulate the behavior and interactions of their physical counterparts, providing valuable insights for diagnosis, treatment, and operational efficiency.
Applications in Healthcare
Digital twins have numerous applications in healthcare. For example, patient-specific digital twins can be used to simulate disease progression and treatment responses, allowing for personalized treatment plans. In medical device manufacturing, digital twins enable predictive maintenance and quality control, reducing downtime and improving patient safety. Moreover, digital twins of hospital systems can optimize resource allocation and workflow, enhancing operational efficiency.
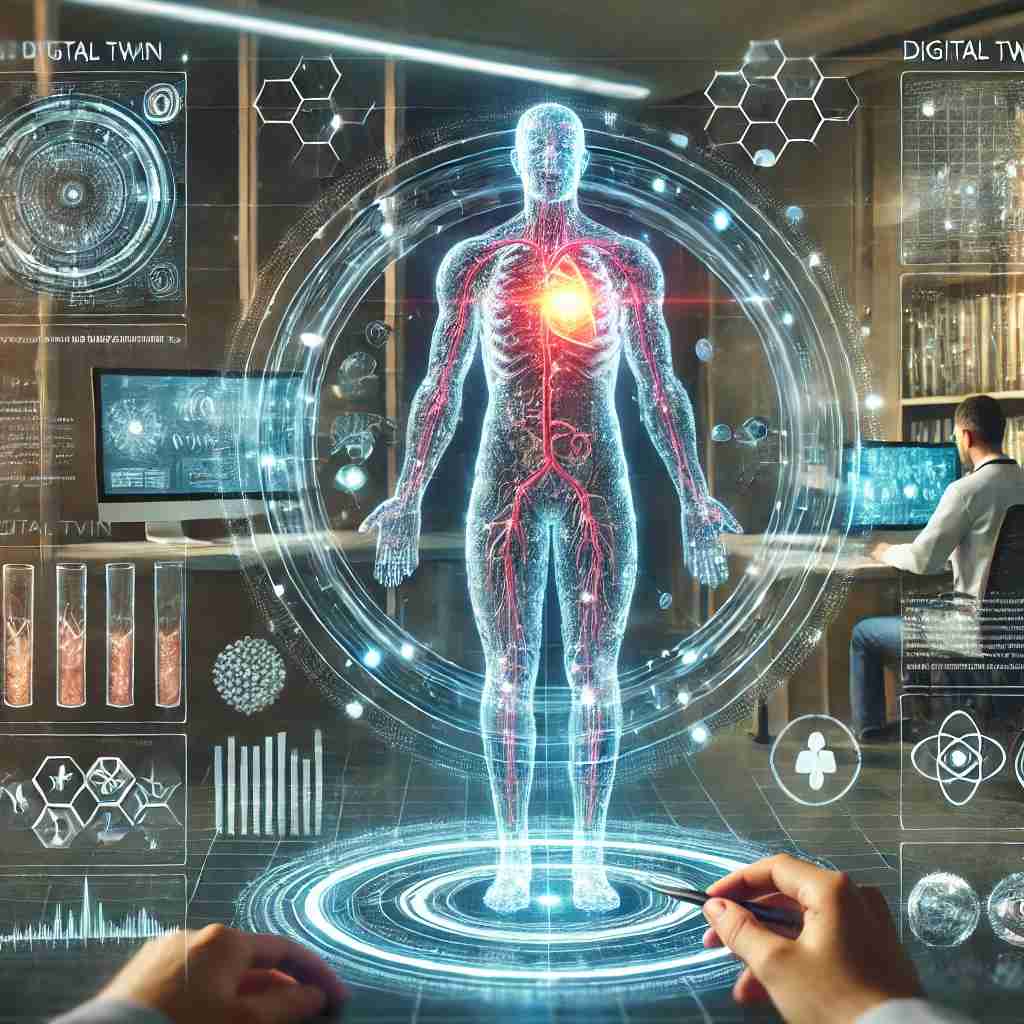
Case Studies and Success Stories
One notable example of digital twin technology in healthcare is the use of patient-specific cardiac models for planning complex heart surgeries. These digital twins simulate the patient’s heart and predict how it will respond to different surgical interventions. This allows surgeons to plan and optimize procedures, reducing risks and improving outcomes. Similarly, digital twins of medical devices like MRI machines enable predictive maintenance, ensuring optimal performance and minimizing downtime.
Challenges and Future Directions
Despite its potential, the implementation of digital twin technology in healthcare faces several challenges. Creating accurate and reliable digital twins requires high-quality data and sophisticated modeling techniques. Additionally, integrating digital twins into existing healthcare workflows and systems can be complex and costly. However, ongoing advancements in data analytics, machine learning, and computational power are likely to overcome these challenges. The future of digital twins in healthcare looks promising, with the potential to revolutionize personalized medicine, medical device management, and hospital operations.
Quantum Computing in Genomics
Understanding Quantum Computing
Quantum computing holds immense potential in genomics, promising to revolutionize the analysis of genetic data. This technology can process complex datasets at unprecedented speeds, facilitating advancements in precision medicine and personalized healthcare. Researchers are exploring how quantum computing can accelerate genomic analysis, potentially leading to breakthroughs in understanding and treating genetic disorders.
Quantum computing leverages the principles of quantum mechanics to perform computations that are infeasible for classical computers. Quantum bits, or qubits, can exist in multiple states simultaneously, enabling parallel processing and exponential increases in computational power. This makes quantum computing particularly well-suited for solving complex problems in genomics.
Applications in Genomics
Genomic data analysis involves processing massive datasets to identify genetic variations and their associations with diseases. Quantum computing can significantly speed up this process, enabling faster and more accurate genomic analysis. This has the potential to accelerate the discovery of disease-causing genes, improve diagnostic accuracy, and facilitate the development of targeted therapies. Additionally, quantum computing can enhance the simulation of molecular interactions, aiding in drug discovery and personalized treatment planning.
Challenges and Ethical Considerations
The application of quantum computing in genomics is still in its early stages, with several challenges to overcome. Quantum computers are currently limited in their qubit capacity and error rates, posing technical hurdles for large-scale genomic analysis. Additionally, the ethical implications of genomic data analysis, such as data privacy and informed consent, need to be carefully addressed. Ensuring that quantum computing advancements are used ethically and responsibly is paramount.
Future Prospects
Despite the challenges, the future of quantum computing in genomics is promising. Ongoing research and development efforts are focused on increasing qubit capacity, reducing error rates, and developing quantum algorithms optimized for genomic analysis. As these advancements continue, quantum computing is poised to revolutionize precision medicine, enabling faster and more accurate genomic insights and ultimately improving patient outcomes.
mRNA Vaccine Technology Beyond COVID-19
How mRNA Vaccines Work
The success of mRNA vaccines in combating COVID-19 has opened new avenues for this technology. Researchers are now exploring its applications in treating other infectious diseases and even cancer. The flexibility and rapid development cycle of mRNA technology make it a promising tool for addressing various health challenges.
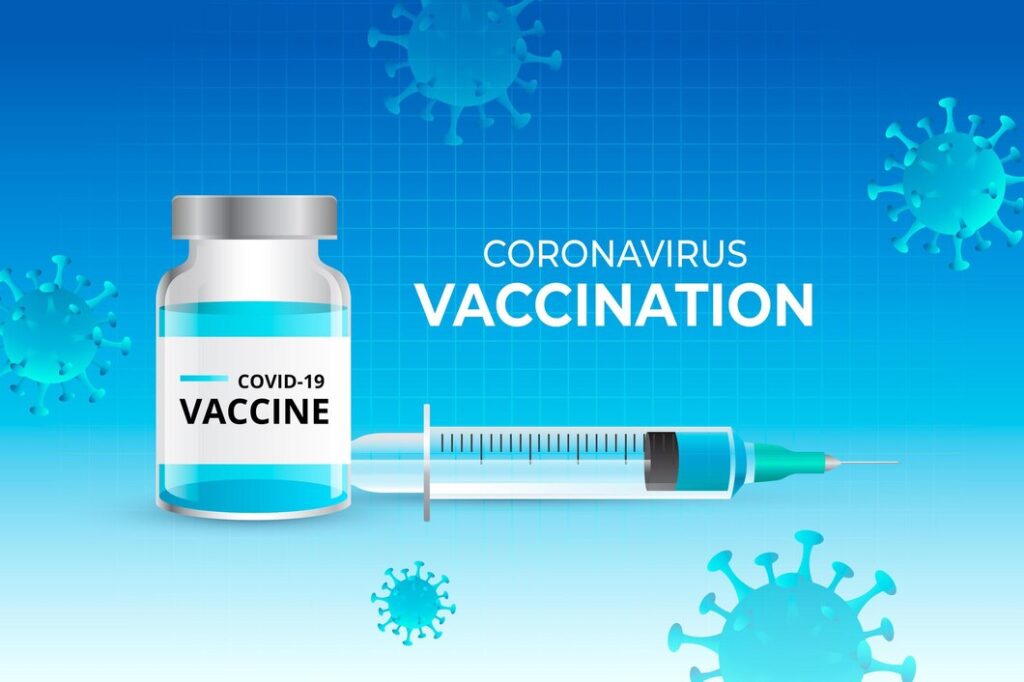
mRNA vaccines work by introducing a small piece of genetic material (mRNA) into the body, which instructs cells to produce a protein found on the surface of a pathogen. This triggers an immune response, preparing the body to recognize and fight the actual pathogen if encountered. Unlike traditional vaccines, which often use inactivated or weakened pathogens, mRNA vaccines do not carry the risk of causing disease and can be developed more quickly.
Beyond COVID-19
The success of mRNA vaccines in the COVID-19 pandemic has spurred interest in their potential applications beyond the virus. Researchers are exploring the use of mRNA technology for developing vaccines against other infectious diseases, such as influenza, Zika virus, and HIV. Additionally, mRNA vaccines are being investigated for their potential in cancer immunotherapy, where they can be designed to target specific cancer cells and stimulate the immune system to attack tumors.
Advantages and Challenges
One of the key advantages of mRNA vaccines is their rapid development cycle. Once the genetic sequence of a pathogen is known, mRNA vaccines can be designed and produced quickly, enabling faster responses to emerging infectious diseases. Additionally, mRNA vaccines can be easily modified to address new variants of a pathogen. However, challenges remain, including the need for ultra-cold storage and distribution infrastructure, which can limit their accessibility in low-resource settings. Ongoing research aims to develop more stable mRNA formulations that can withstand standard refrigeration conditions.
The Road Ahead
The future of mRNA vaccine technology is bright, with ongoing advancements in vaccine design, manufacturing, and delivery. The lessons learned from the COVID-19 pandemic have paved the way for the rapid development and deployment of mRNA vaccines for other diseases. Continued investment in research and infrastructure will be essential in realizing the full potential of this transformative technology, ultimately leading to improved global health outcomes.
Remote Patient Monitoring Systems
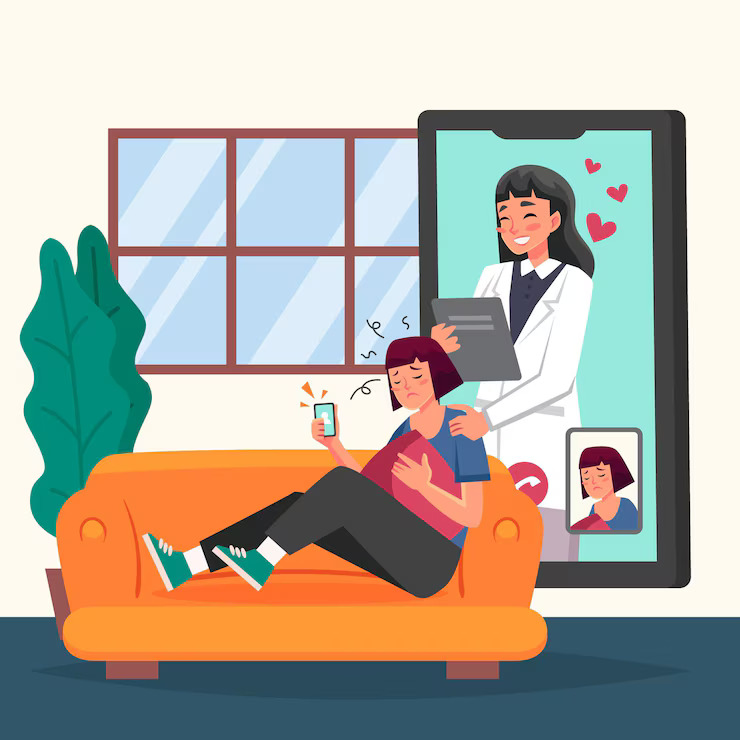
Understanding Remote Patient Monitoring
Advancements in IoT and wearable technology are driving the adoption of remote patient monitoring systems. These systems allow continuous tracking of patients’ health metrics, enabling early intervention and improved chronic disease management. Wearable devices equipped with sensors collect real-time data, providing healthcare providers with valuable insights into patients’ health status.
Remote patient monitoring (RPM) involves the use of connected devices to monitor patients’ health conditions outside of traditional healthcare settings. These devices collect data on various health metrics, such as heart rate, blood pressure, glucose levels, and physical activity, and transmit it to healthcare providers in real-time. RPM enables continuous monitoring and early detection of health issues, allowing for timely interventions and reducing the need for hospital visits.
Applications and Benefits
RPM is particularly beneficial for managing chronic diseases such as diabetes, hypertension, and heart failure. By providing real-time insights into patients’ health, RPM helps healthcare providers make informed decisions and adjust treatment plans as needed. This proactive approach can prevent complications, improve patient outcomes, and reduce healthcare costs. Additionally, RPM enhances patient engagement and empowerment by involving them in their own care and encouraging adherence to treatment plans.
Case Studies and Success Stories
Numerous case studies highlight the effectiveness of RPM in improving patient outcomes. For example, a study conducted by the Veterans Health Administration (VHA) found that RPM reduced hospital admissions and emergency room visits among veterans with chronic conditions. Another example is the use of wearable devices for monitoring heart failure patients, which has been shown to reduce hospital readmissions and improve quality of life. These success stories demonstrate the potential of RPM to transform chronic disease management and improve patient care.
Challenges and Future Directions
Despite its benefits, the adoption of RPM faces several challenges. One major challenge is data security and privacy, as RPM involves the collection and transmission of sensitive health information. Ensuring robust cybersecurity measures and compliance with data protection regulations is crucial. Additionally, integrating RPM into existing healthcare workflows and systems can be complex and requires adequate training and support for healthcare providers. However, ongoing advancements in IoT, wearable technology, and data analytics are likely to overcome these challenges. The future of RPM looks promising, with the potential to revolutionize chronic disease management and enhance patient care.
Wearable Tech for Chronic Disease Management
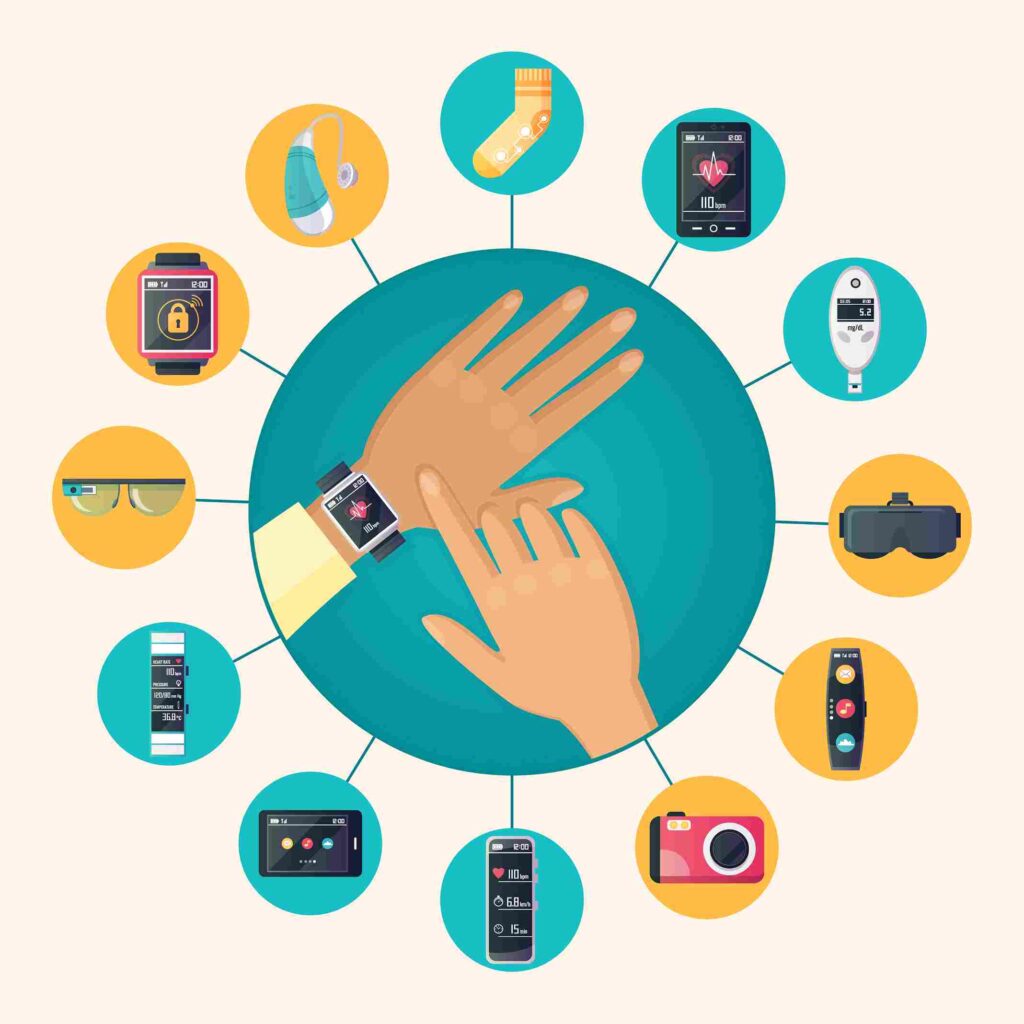
The Rise of Wearable Technology
Wearable technology is playing a crucial role in managing chronic diseases like diabetes and cardiovascular conditions. Devices such as smartwatches and fitness trackers monitor vital signs, activity levels, and other health metrics, empowering patients to take a proactive approach to their health. These wearables also facilitate better communication between patients and healthcare providers, ensuring timely interventions.
Wearable technology has gained popularity in recent years, with devices like smartwatches, fitness trackers, and continuous glucose monitors becoming increasingly common. These devices are equipped with sensors that collect data on various health metrics, such as heart rate, physical activity, sleep patterns, and glucose levels. The data is then analyzed and presented to users in real-time, providing valuable insights into their health.
Applications in Chronic Disease Management
Wearable technology is particularly beneficial for managing chronic diseases. For example, continuous glucose monitors (CGMs) help individuals with diabetes monitor their blood glucose levels in real-time, enabling better glycemic control and reducing the risk of complications. Similarly, smartwatches and fitness trackers monitor physical activity and heart rate, helping individuals with cardiovascular conditions track their exercise and detect irregularities. Wearable devices also facilitate remote patient monitoring, allowing healthcare providers to monitor patients’ health and make timely interventions.
Case Studies and Success Stories
Numerous studies and real-world examples highlight the effectiveness of wearable technology in managing chronic diseases. For instance, a study published in the Journal of Medical Internet Research found that the use of CGMs improved glycemic control and reduced the incidence of hypoglycemia in individuals with type 1 diabetes. Another example is the use of smartwatches for monitoring heart failure patients, which has been shown to reduce hospital readmissions and improve quality of life. These success stories demonstrate the potential of wearable technology to improve chronic disease management and patient outcomes.
Challenges and Future Directions
Despite its benefits, the widespread adoption of wearable technology for chronic disease management faces several challenges. One major challenge is data privacy and security, as wearable devices collect and transmit sensitive health information. Ensuring robust cybersecurity measures and compliance with data protection regulations is crucial. Additionally, integrating wearable technology into existing healthcare systems and workflows can be complex and requires adequate training and support for healthcare providers. However, ongoing advancements in wearable technology and data analytics are likely to overcome these challenges. The future of wearable technology in chronic disease management looks promising, with the potential to empower patients, improve outcomes, and reduce healthcare costs.
Ethical Implications of AI in Clinical Decision-Making
The Role of AI in Clinical Decision-Making
As AI becomes more integrated into clinical decision-making, ethical considerations are gaining prominence. Balancing the benefits of AI with the need for patient privacy and autonomy is a critical challenge. Healthcare providers and policymakers must address concerns related to bias, transparency, and accountability to ensure the ethical use of AI in healthcare.
AI has the potential to transform clinical decision-making by analyzing vast amounts of data and providing evidence-based recommendations. AI algorithms can assist in diagnosing diseases, predicting patient outcomes, and recommending treatment plans. This has the potential to improve diagnostic accuracy, enhance treatment effectiveness, and reduce healthcare costs.
Ethical Considerations
The integration of AI into clinical decision-making raises several ethical considerations. One major concern is bias in AI algorithms. If the training data used to develop AI models is biased, the resulting algorithms may perpetuate existing disparities in healthcare. Ensuring that AI models are trained on diverse and representative data is crucial to mitigate bias. Additionally, transparency is a significant concern, as AI algorithms are often considered “black boxes” with opaque decision-making processes. Ensuring that AI models are transparent and explainable is essential for gaining the trust of healthcare providers and patients.
Accountability and Responsibility
Another ethical consideration is accountability and responsibility for AI-driven decisions. If an AI algorithm makes an incorrect diagnosis or treatment recommendation, it is essential to determine who is responsible for the error—the healthcare provider, the AI developer, or the institution using the AI system. Establishing clear guidelines and regulations for accountability is crucial to ensure that AI is used responsibly in healthcare.
The Road Ahead
Addressing the ethical implications of AI in clinical decision-making requires a collaborative effort from healthcare providers, policymakers, AI developers, and ethicists. Developing robust ethical frameworks and guidelines for the use of AI in healthcare is essential. This includes ensuring data privacy and security, mitigating bias, enhancing transparency, and establishing clear accountability measures. The future of AI in clinical decision-making holds great promise, but it must be guided by strong ethical principles to ensure that it benefits all patients and improves healthcare outcomes.
Smart Hospitals and AI Integration
What Are Smart Hospitals?
The concept of smart hospitals, where AI and other advanced technologies are integrated into healthcare infrastructure, is gaining traction. These hospitals use AI for various applications, including patient management, predictive maintenance of medical equipment, and optimizing hospital operations. The integration of AI enhances efficiency, reduces costs, and improves patient outcomes.
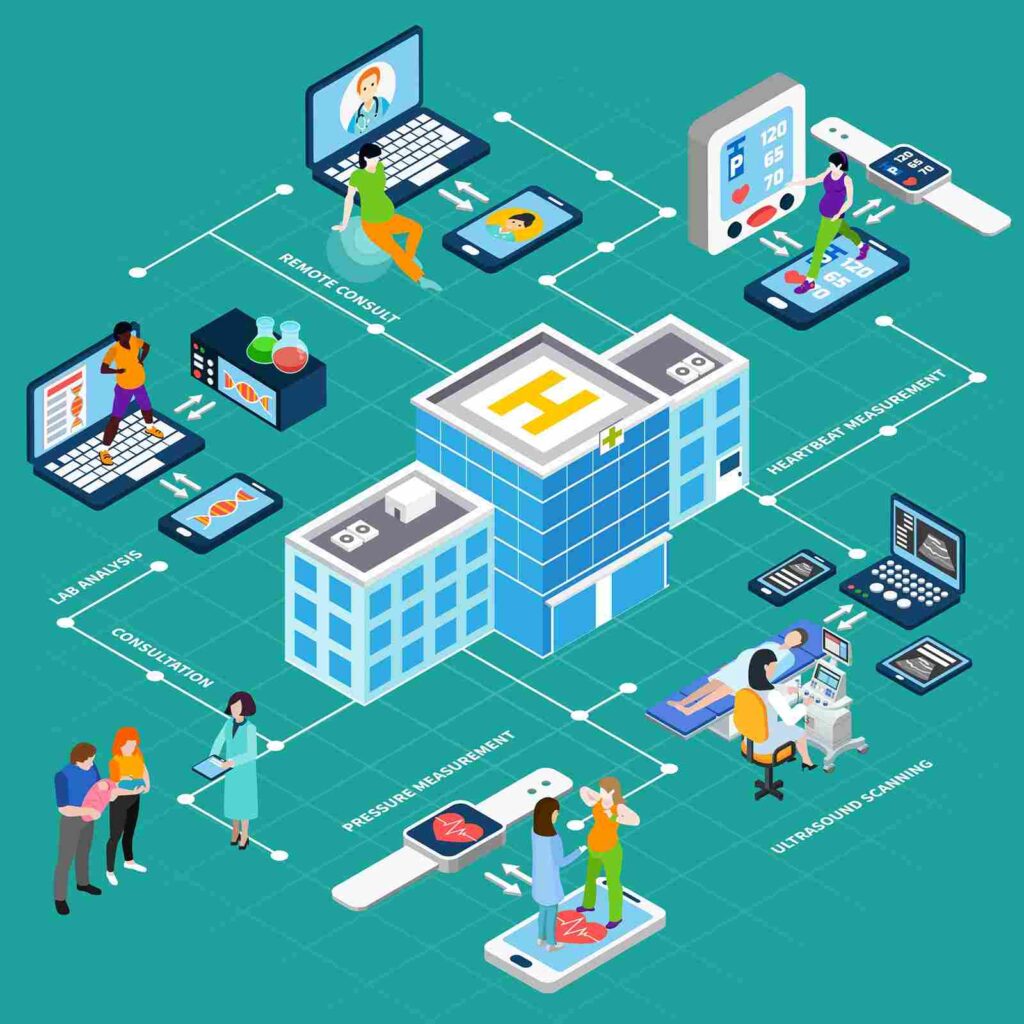
Smart hospitals leverage advanced technologies, including AI, IoT, and big data analytics, to optimize healthcare delivery and improve patient outcomes. These hospitals use interconnected systems to collect, analyze, and share data in real-time, enabling more efficient and effective healthcare operations. AI plays a central role in smart hospitals, providing predictive analytics, automation, and decision support.
Applications and Benefits
Smart hospitals use AI for various applications, including patient management, predictive maintenance of medical equipment, and optimizing hospital operations. For example, AI-powered patient management systems can analyze patient data to predict admissions, optimize bed allocation, and streamline discharge processes. Predictive maintenance algorithms can monitor medical equipment and predict failures before they occur, reducing downtime and ensuring optimal performance. Additionally, AI can optimize hospital operations by analyzing workflow data and identifying inefficiencies, leading to improved resource allocation and reduced costs.
Case Studies and Success Stories
Numerous smart hospitals around the world are demonstrating the benefits of AI integration. For example, the Mayo Clinic in the United States uses AI-powered predictive analytics to optimize patient flow and reduce wait times. Similarly, Mount Sinai Hospital in New York uses AI to predict patient deterioration and guide early interventions, improving patient outcomes. These success stories highlight the potential of smart hospitals to enhance healthcare delivery and improve patient care.
Challenges and Future Directions
Despite the benefits, the implementation of smart hospital technology faces several challenges. One major challenge is the high cost of infrastructure and technology integration, which can be a barrier for many healthcare institutions. Additionally, ensuring data privacy and security is crucial, as smart hospitals rely on the collection and analysis of sensitive patient data. Finally, the integration of AI and other advanced technologies into existing healthcare workflows and systems can be complex and requires adequate training and support for healthcare providers. However, ongoing advancements in technology and the increasing demand for efficient healthcare delivery are likely to drive the adoption of smart hospital solutions. The future of smart hospitals looks promising, with the potential to transform healthcare delivery and improve patient outcomes.
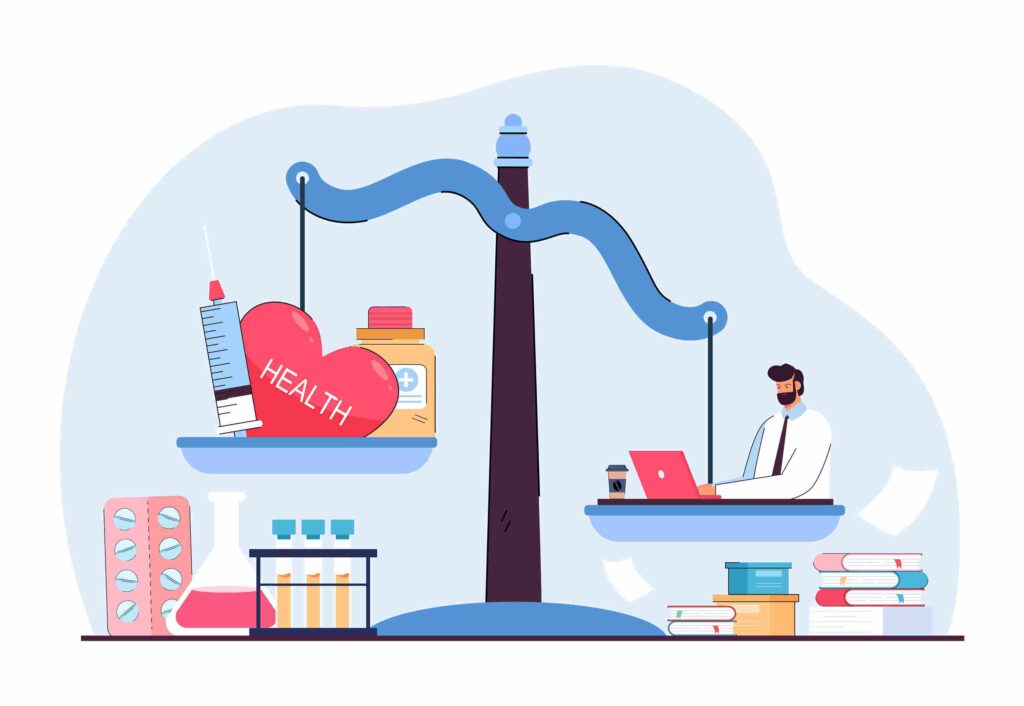
Mental Health Apps and Digital Therapeutics
The Role of Digital Health in Mental Health
Digital health solutions for mental health are expanding, with apps and digital therapeutics offering accessible and effective treatments. These tools provide cognitive behavioral therapy, mindfulness exercises, and other interventions through mobile platforms. The rise of mental health apps addresses the growing demand for mental health services, particularly in areas with limited access to traditional care.
Digital health solutions, including mental health apps and digital therapeutics, are playing an increasingly important role in mental health care. These tools provide convenient and accessible interventions for individuals seeking mental health support. Mental health apps offer a range of features, including cognitive behavioral therapy (CBT), mindfulness exercises, mood tracking, and peer support. Digital therapeutics, on the other hand, are evidence-based interventions delivered through digital platforms and prescribed by healthcare providers.
Applications and Benefits
Mental health apps and digital therapeutics offer several benefits. They provide individuals with convenient and accessible tools to manage their mental health, reducing barriers to care. These tools are particularly valuable in areas with limited access to mental health professionals, such as rural or underserved communities. Additionally, mental health apps can enhance engagement and adherence to treatment plans, providing real-time support and tracking progress.
As we navigate through 2024, the transformative power of advanced technologies in healthcare becomes increasingly evident. Generative AI, quantum computing, and other cutting-edge innovations are not only enhancing the capabilities of medical professionals but are also empowering patients with more personalized and efficient care. These advancements promise to tackle some of the most pressing challenges in healthcare, from accelerating drug discovery to enabling early disease detection and more precise treatments. However, the integration of these technologies also brings forth challenges such as ensuring data privacy, addressing ethical concerns, and managing the cost of implementation. Despite these hurdles, the potential benefits far outweigh the obstacles, heralding a new era of healthcare that is more responsive, predictive, and patient-centric. As the industry continues to evolve, ongoing collaboration between technology developers, healthcare providers, and policymakers will be crucial in harnessing these innovations to their fullest potential, ultimately leading to a healthier future for all.
Authors Biography
Mercilina Norman, is currently pursuing an MBA in healthcare and hospital administration. She also has experience as a staff nurse.