Artificial Intelligence (AI) is a hot topic, simply put – it’s a way of making a computer think intelligently, in a way human think and over a decade now it has managed to be fairly successful. It has found application in several domains, from consumer electronics like smartphones and smart home devices like Amazon’s Alexa to very niche applications in academic research. What began as a nascent academic pursuit to enable computers to think and solve problems using human-like cognitive capabilities has now invaded most aspects of human life, medicine and healthcare is no exception.
Modern medicine has discovered around 60,000 ways things can go wrong with the human body and over thousands of years have probed these illnesses and disorders to better understand and treat them, one drug, one technique at a time. In recent years, however, there has been a dramatic shift in the pace of innovation in healthcare, especially with the advent of artificial intelligence. Artificial Intelligence is an umbrella term used to cover a wide array of algorithms which mimic human cognitive functions and are self-correcting, and can ‘learn’ from a dataset.
A mountain of unstructured data
One area where AI would do heaps of help to physicians and medical practitioners is to deal with the insurmountable amount of clinical unstructured data. Nearly 80% of the clinical information is “unstructured” and in a format incomprehensible to health information systems. Thus, getting useful information from these so-called unstructured databases becomes a labor-intensive task. To top that, clinical data is doubling every three years; which leaves the healthcare system with a massive volume of unsorted heterogeneous patient information which may hold answers to several health challenges, but strictly speaking, is of little use in its current form. This challenge in healthcare of too much data, too little insight can be alleviated by employing Natural Language Processing (NLP), a form of AI which identifies key information from spoken or written human input, such as physical examination records, handwritten lab notes, discharge summaries etc. The promise of NLP lies in its ability to turn this big data into smart data. It can be applied to mine big blocks of clinical data and convert that into organized curated easy-for-retrieval information, which can make documentation of clinical information more manageable. In 2014, IBM’s Watson collaborated with Epic Systems and Carilion Clinic to analyze massive 21 million records in just six weeks and pulled important information about risk factors and other features from examination notes written by physicians and clinical laboratory results into organized EHR templates, and further used predictive modeling to identify patients at risk to congestive heart failure with an assuring 85% accuracy rate. Similar efforts of using NLP to tackle cancer and genomics datasets are in process. NLP algorithms thus can be employed with much effectiveness to unlock healthcare’s big data crisis to extract clinically relevant information and make it available for doctors to make smart decisions about their patients.
Also Read: Medical Devices In India Witness Churning
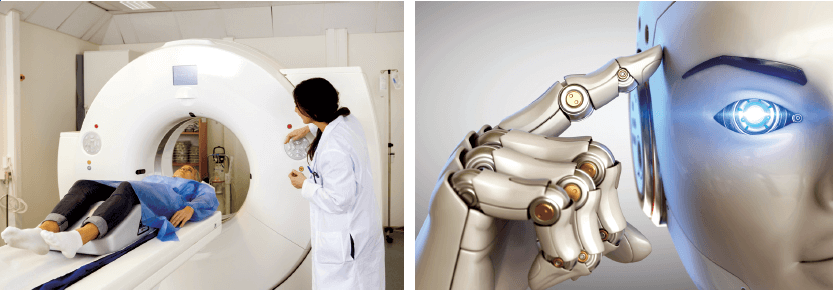
Can Artificial Intelligence replace a doctor?
Another facet of healthcare where artificial intelligence can find use is analyzing structured data namely genetic data, imaging data from X-ray scans, CT scans, MRIs, etc. and electrophysiological data obtained from electrography of the heart, brain, and other body parts. Machine learning plays a major role owing to its ability to ‘learn’ and make predictions from data without explicit programming. Of the many machine learning algorithms, two such algorithms have been used extensively in both research and healthcare, namely Support Vector Machine (SVM) and Neural Networks (NN), both use supervised learning models. SVM, in particular, has been useful in tasks involving classification and for novelty detection. For example, a 2012 study used SVM to identify imaging biomarkers of neurological and psychiatric disease. SVM has been used as prediction models for diabetic and prediabetic patients. In 2010, a research group from Korea applied SVM to make predictions about heart failure patients and their adherence rate to their medication. Two researchers from Australia used SVM for the diagnosis of cerebral palsy gait with an accuracy rate of 96.8%.
Neural Networks, on the other hand, form another major chunk of AI algorithm in healthcare. NN algorithms are vaguely based on biological neural networks, in which a collection of interconnected nodes processes the data like how neurons communicate in a human brain. The potential of NN has been multiplied manifold, thanks to the advent of Deep Learning which is an evolved form of NN, it uses multiple hidden layers that can be used to process complex multidimensional data like a human brain. A huge portion of NN algorithms is used for diagnostic imaging. Early last year, a study published in Nature used CNN, a type of deep learning NN algorithm to identify skin cancer from clinical images. The algorithm which was trained on 29,450 clinical images, was highly specific and sensitive to detection and was on par with the performance of an expert dermatologist with over 90% accuracy. A 2016 study used a variant of deep learning NN to identify interstitial lung disease using CT scan images with 85.5% accuracy. Google’s artificial intelligence team employed deep learning algorithms to study pictures of the back of the eye, for the detection of diabetic retinopathy, a blinding disorder in diabetic patients. Their results showed above 90% accuracy in both sensitivity and specificity of detection, which is at par with a skilled ophthalmologist.
Also Read: Advantages & Disadvantages: Real Time Health Monitoring Devices
Some areas where artificial intelligence surpasses humans is in looking for patterns in data and in making predictions about that data. Processing thousands of images and looking for a subtle discernible pattern within huge volumes of data is a tough task for humans, but that’s what Shinjini Kundu, a physician at the University of Pittsburgh Medical Center has been doing. Her AI algorithms examine images like MRI scans for subtle differences which may not be perceptible to the human eye, and she has employed this to study osteoarthritis and to predict its development way before it’s diagnosis with a whopping 86.2% accuracy. Similar algorithms can be used to see nuanced differences in electrocardiograms, CT scan images and even in oncology to look for invisible patterns of disease onset and progression. As artificial intelligence algorithms get better after each iteration, routine lab tests like X-rays, CT scans, MRI scans, ECG etc. would fall into the domain of artificial intelligence for more quick and reliable results.
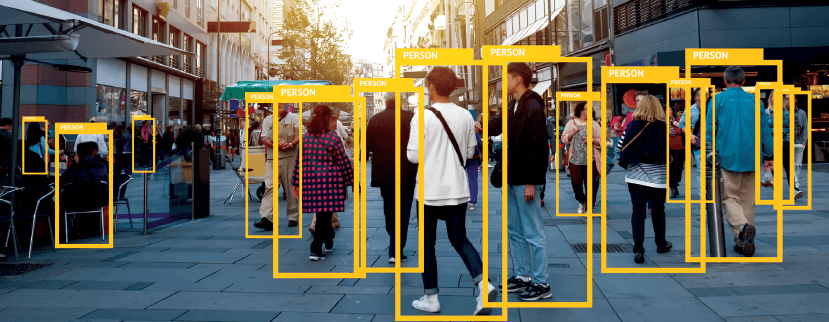
Investment in AI-centered healthcare
Beyond research laboratories and hospitals, the emergence of AI has caused exponential growth in policies regarding AI and investment in AI around the world. AI-based startups have seen rampant growth. Startup Health, an incubator in the US recently reported that there were 7,600 healthcare start-ups around the world working on digital health innovation, a major portion of which involves AI based innovation. An Accenture report published in late 2017 states, “Growth in the AI health market is expected to reach $6.6 billion by 2021 – that’s a compound annual growth rate of 40%”. Another report by CIS India published this year states that AI could add a whopping $957 billion to the Indian economy by 2035. Even state governments are pushing for growth in AI-based sectors. The government of India aims to increase healthcare spending to 2.5% of the Gross Domestic Product (GDP) by the end of its 12th five-year plan, and to 3% by 2022. Such high rates of adoption are due to several AI start-ups and involvement of major players like Microsoft and IBM.
Given the skewed ratio of doctors to patients in India, AI-based healthcare techniques would provide much-needed help in providing healthcare amenities to the masses. Globally, US government have made heavy investments in two of its AI-centered healthcare initiatives, with $1 billion proposed budget to its Cancer Moonshot Program and another $215 million in its Precision Medicine Initiative.
Ethics and issues with AI in healthcare
As rapidly as AI has been embraced by the medical and healthcare community, its benefits cannot be actualized without understanding its ethical pitfalls. But there are several concerns when applying these algorithms at a large scale to make real clinical decisions. Algorithms, albeit self-learning is products designed by human and may reflect their biases in the results they produce. These algorithms may reflect the biases of its designer or biases caused by the dataset on which the algorithm was trained. For example, algorithms developed by private sector entities can be biased to ensure outcomes of their interest or healthcare institutes may use AI systems selectively based on say, insurance plan or economic status of that patient or any other parameter.
Even though Deep Learning algorithms can perform sophisticated predictions on imaging data, they are essentially not fed by an explicit code of information but are self-taught systems and even though the prediction score it gives, for example, whether the lesion is malignant or benign are surprisingly accurate when corroborated with the diagnostic report by a doctor, there’s no way to determine how exactly it came to that conclusion, thus rendering AI systems as a black box; with little clarity on how it works. Recently though there have been several predictions to understand how deep learning works, the information bottleneck theory being a prominent one, but the debate is far from settled.
The issues mentioned above are all pertaining to the AI system and its functioning, but there are vital concerns about AI’s effect on people involved in care. Several studies have shown that patients prefer AI chatbots and virtual nurses over humans when learning about their diagnosis as they can proceed to learn at their own pace without the embarrassment of not keeping up with the doctor’s speed. Patients are also more open to conversation with a computer than a human being, part of the reason being the diminished shame and fear associated with being vulnerable. But Allison Pugh, a Professor of Sociology at the University of Virginia and a writer for the New Yorker, thinks that virtual nurses and AI bots offer nothing more than the thinnest veil of care. She writes, “[…] automating or using AI to deliver care would be the same as relying on a “cloth monkey”—a reference to a cruel experiment, carried out in 1959, in which infant monkeys were given a choice between two surrogate mothers, one made from welded wire, the other from terry cloth. (The infants preferred the cloth mother, even when only the wire mother gave them milk.) AI-driven care was a sorry version of the real thing.”
As demonstrated by several research groups, deep learning algorithms have achieved human-level accuracy and then some more. It can look for patterns which are invisible to the human eye. Thus, sooner or later, displacing and relegating doctors from their positions, at least in certain areas of healthcare. This can lead to massive burnouts in doctors as their roles shift drastically and may even lead to a gradual attrition of their skills. But there’s more to care than just interpreting blood reports and imaging data of a patient, it has much more to do about understanding the needs of patients, their mental state, etc. The secret of healthcare is not in reading out objective reports, but in the assurance and the warmth, a doctor’s cadence can provide. “Caring is expressed in listening, in the time-honored ritual of the skilled bedside exam – reading the body – in touching and looking at where it hurts and ultimately in localizing the disease for patients not on a screen, not on an image, not on a biopsy report, but on their bodies.”, writes Abraham Verghese, an author and a physician at Stanford.
Employing AI to most healthcare activities might also have a negative effect on how knowledge is generated. Most medical knowledge generated in the past has been curiosity driven. AI systems can tell us whether the lesion is a benign mole or a tumor, but it can’t provide answers to why the tumor has a corrugated surface or white patches etc.
Artificial intelligence is going to be pervasive across the spectrum of healthcare. From routine lab tests to offering a clinical decision, AI algorithms will play a major role in the future of healthcare. As deep learning algorithms get stronger and as the workings of the black box are revealed, AI technology will make further strides in healthcare. But advancements in AI-based healthcare doesn’t mean the downfall of human doctors. Healthcare is a highly emotional and human-centric field and the “human touch” will always play a pivotal role in the delivery of healthcare. Humans, even highly skilled doctors are fallible beings with inherent limitations and artificial intelligence will not sideline these practitioners but augment their abilities, in making an objectively better yet humane decision.
About the author
Pratik Pawar is a science writer based in Mumbai. He has a Master’s degree in Biotechnology and currently works as a freelancer writing science-centric pieces with a focus on neuroscience.
Pictures credit: InnoHEALTH Magazine