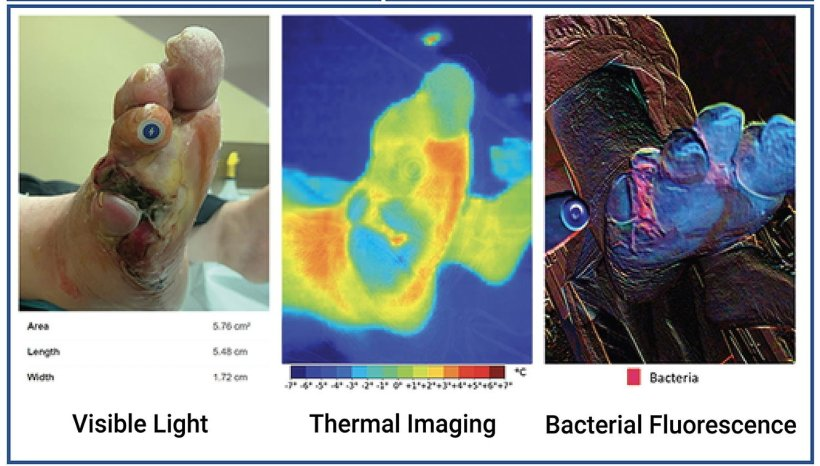
Source: frontiers
Wound care today is one of the most expensive and overlooked threats to patients and our overall healthcare system. It is very difficult for doctors to identify a wound which is becoming infected. Clinical signs and symptoms are imprecise and methods of identifying bacteria can be inaccessible or time-consuming. Keeping this in mind, an International team of Researchers have deduces a solution in the form of ‘SWIFT RAY 1’ which is a device run from a smart phone or tablet application that allows advanced imaging of a wound to identify infection.
This device can be attached to a smart phone and connected to the Swift Skin and Wound software to take medical-grade photographs, bacterial fluorescence images (which reveal bacteria using violet light) and infrared thermography images (which measure body heat). By capturing the heat produced by a wound and the fluorescence of bacteria, it helps clinicians tell the difference between inflammation and infection. This could help in faster intervention by catching infections before they pose serious threat to the patient.
Testing of the device was done by recruiting 66 wounded patients. Their wounds showed no sign of infection spreading further, did not contain foreign bodies and had not previously been treated with antibiotic or growth factors. The wounds were cleaned, uncovered and dried before imaging and later cared for as usual.
A researcher who was not present for the wound care process, reviewed the images which identified four patterns. ‘Non-inflamed’ wounds were the ones where the wound was not warmer than healthy skin and no bacterial flouroscence was present. ‘Inflamed’ wounds were the ones which were slightly warmer than healthy skin and no or slight bacterial fluoroscence. Last two patterns of wounds which were substantially warmer with or without bacterial fluorescence were both designated as ‘Infected’ because all the clinicians who had examined these wounds has considered them infected. So out of the 66 wounds, 20 were non-inflamed, 26 were inflamed and 20 were infected.
The researchers performed principal component analysis and used an algorithm called k-neighbor clustering to see if a machine learning model could accurately identify these different categories of wound. It was found out that the model could identify all three very well with an accuracy of 74%. When differentiating between infected and non-infected wounds, the model correctly identified 100% of infected wounds and 91% of non-infected wounds.
This was a pilot study and follow-up studies are planned. This research has demonstrated bacterial imaging helps guide clinicians work to remove nonviable tissue, yet it cannot identify infection by itself and also that the images should always be considered in their medical context. The research paper is published in Frontiers in Medicine.